Document Type
Conference Proceeding
Original Publication Date
2015
Journal/Book/Conference Title
14th INFORMS Computing Society Conference
First Page
226
Last Page
235
DOI
10.1287/ics.2015.0017
Date of Submission
February 2015
Abstract
The support vector machine (SVM) is a flexible classification method that accommodates a kernel trick to learn nonlinear decision rules. The traditional formulation as an optimization problem is a quadratic program. In efforts to reduce computational complexity, some have proposed using an L1-norm regularization to create a linear program (LP). In other efforts aimed at increasing the robustness to outliers, investigators have proposed using the ramp loss which results in what may be expressed as a quadratic integer programming problem (QIP). In this paper, we consider combining these ideas for ramp loss SVM with L1-norm regularization. The result is four formulations for SVM that each may be expressed as a mixed integer linear program (MILP). We observe that ramp loss SVM with L1-norm regularization provides robustness to outliers with the linear kernel. We investigate the time required to find good solutions to the various formulations using a branch and bound solver.
Rights
Creative Commons Attribution 3.0 Unported (CC BY 3.0)
Is Part Of
VCU Statistical Sciences and Operations Research Publications
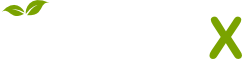
- Citations
- Citation Indexes: 4
- Usage
- Downloads: 14035
- Abstract Views: 1234
- Captures
- Readers: 236
Comments
Originally published at http://dx.doi.org/10.1287/ics.2015.0017.
Creative Commons Attribution 3.0 Unported License (CC BY 3.0)
Accompanying data files available at http://scholarscompass.vcu.edu/ssor_data/1/.