Document Type
Conference Proceeding
Original Publication Date
2015
Journal/Book/Conference Title
14th INFORMS Computing Society Conference
First Page
212
Last Page
225
DOI
10.1287/ics.2015.0016
Date of Submission
February 2015
Rights
Creative Commons Attribution 3.0 Unported (CC BY 3.0)
Recommended Citation
Principal component analysis (PCA) is one of the most widely used multivariate techniques in statistics. It is commonly used to reduce the dimensionality of data in order to examine its underlying structure and the covariance/correlation structure of a set of variables. While singular value decomposition provides a simple means for identification of the principal components (PCs) for classical PCA, solutions achieved in this manner may not possess certain desirable properties including robustness, smoothness, and sparsity. In this paper, we present several optimization problems related to PCA by considering various geometric perspectives. New techniques for PCA can be developed by altering the optimization problems to which principal component loadings are the optimal solutions.
Is Part Of
VCU Statistical Sciences and Operations Research Publications
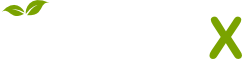
- Citations
- Citation Indexes: 4
- Usage
- Downloads: 14035
- Abstract Views: 1234
- Captures
- Readers: 236
Comments
Originally published at http://dx.doi.org/10.1287/ics.2015.0016.
Creative Commons Attribution 3.0 Unported License (CC BY 3.0)
Accompanying R Code data files available at http://scholarscompass.vcu.edu/ssor_data/2/.